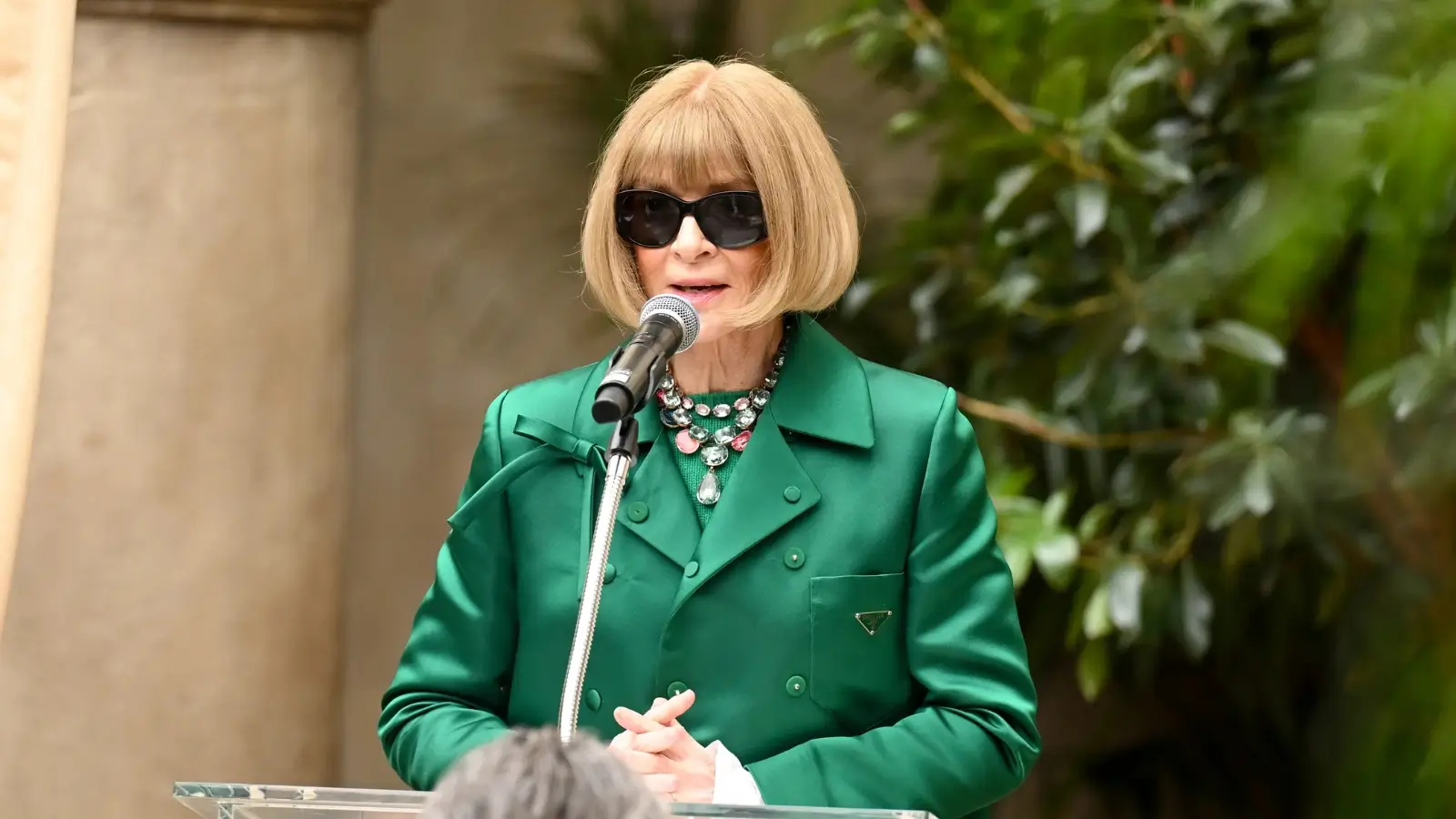

Predictive analytics has become an indispensable tool in modern business, transforming how companies understand and engage with their customers. By leveraging historical data, machine learning algorithms, and behavioral patterns, predictive models forecast customer needs and identify potential risks, enabling businesses to make proactive decisions. These models play a pivotal role in improving customer retention strategies, a cornerstone of sustainable growth.
Retaining existing customers is significantly more cost-effective than acquiring new ones. Studies indicate that acquiring a new customer can cost five times more than retaining an existing one. Moreover, increasing customer retention rates by just 5% can boost profits by 25% to 95% through increased loyalty and repeat purchases. Predictive analytics in retail provides the actionable insights needed to prioritize retention, anticipate customer churn, and personalize engagement.
How can companies leverage predictive analytics to secure customer loyalty and build long-term relationships? Let’s explore.
Customer retention is a crucial aspect of sustainable business growth, and identifying at-risk customers early can significantly reduce churn. By leveraging data insights, businesses can pinpoint key indicators of potential disengagement and take proactive measures to re-engage these customers.
Several behaviors and metrics signal that a customer might be on the verge of churn. Reduced engagement, such as a decline in email opens, website visits, or app interactions, is a common early warning. Similarly, a drop in purchase frequency or average order value over time can indicate waning interest. Negative feedback, whether through surveys or reviews, also serves as a red flag that a customer’s experience may not meet their expectations.
To accurately identify at-risk customers, businesses analyze a range of data:
Purchase History: Declines in purchase frequency or total spending can signify a shift in loyalty.
Customer Feedback: Surveys, support tickets, and reviews provide direct insight into customer satisfaction.
Behavioral Patterns: Tracking metrics such as browsing history, abandoned carts, and login frequency can reveal disengagement trends.
Demographics and Preferences: Cross-referencing personal data with market trends can help identify customers who are more likely to churn based on evolving needs.
Advanced predictive algorithms play a pivotal role in flagging at-risk customers. Machine learning models, for instance, can analyze historical and real-time data to assign churn probabilities to individual customers. Examples include:
Decision Trees: These models segment customers based on key attributes, identifying which combinations correlate with churn.
Neural Networks: These models recognize complex patterns in customer behavior that may go unnoticed in traditional analyses.
Clustering Algorithms: By grouping customers with similar behaviors, these algorithms can highlight segments prone to disengagement.
Proactive retention strategies leverage advanced analytics to understand customer behavior, predict churn, and implement timely interventions to enhance loyalty. By using data-driven approaches, businesses can not only mitigate potential revenue loss but also create more meaningful connections with their audience.
One of the most impactful ways to retain customers is through personalized offers tailored to their preferences. Analytics tools process historical purchase data, browsing patterns, and demographic information to identify what resonates with individual customers. Predictive insights go a step further by forecasting future needs, enabling businesses to offer relevant products or services proactively. For instance, a retail brand might analyze seasonal buying patterns to send customized discounts on winter wear to customers who frequently purchase during colder months. Such targeted efforts make customers feel valued, enhancing their loyalty to the brand.
Analytics also helps determine the most effective times and channels for customer outreach. Whether through email, SMS, or app notifications, businesses can optimize engagement by reaching customers when they are most likely to respond. For example, predictive models can analyze past interactions to identify patterns, such as increased engagement during weekend evenings or through mobile app alerts. By aligning outreach efforts with these insights, businesses increase the likelihood of conversions and reduce the risk of appearing intrusive.
Proactive retention strategies have seen significant success in the retail industry. For instance, an eCommerce platform used predictive analytics to identify high-value customers at risk of churn. By offering personalized discounts via their preferred communication channels, the platform reduced churn rates by 25%. Similarly, a global retail chain implemented a loyalty program powered by analytics, which tracked customer purchases and rewarded points for recurring engagements. This initiative not only improved retention but also boosted average customer spend by 15%.
When integrated effectively, analytics-backed retention strategies transform the way businesses interact with their customers. By focusing on personalization, optimal timing, and proven use cases, companies can achieve sustainable growth while building long-term customer relationships. These strategies represent a forward-thinking approach to customer retention in an increasingly data-driven world.
Continuous improvement is a critical aspect of modern business strategies, and analytics serves as the backbone of this evolution. By systematically monitoring, refining, and adapting retention campaigns and customer engagement strategies, businesses can achieve sustainable growth and long-term loyalty. Here’s how analytics powers this process:
The first step in leveraging analytics for continuous improvement is assessing the performance of retention efforts. Key performance indicators (KPIs) like customer churn rates, repeat purchase frequency, and lifetime value (CLV) provide measurable insights into the success of campaigns. Advanced analytics platforms enable businesses to track these metrics in real-time, identifying patterns and trends that highlight areas of improvement. For example, if a subscription service sees a spike in cancellations after promotional offers end, analytics can pinpoint the reasons, such as dissatisfaction with pricing or perceived value.
Predictive analytics elevates retention efforts by providing foresight into customer behavior. By analyzing historical data and behavioral patterns, businesses can predict potential churn and take proactive measures. For instance, a retailer might use predictive models to identify customers likely to stop shopping with them and deploy personalized offers or exclusive loyalty rewards to re-engage them. Over time, these insights allow for the optimization of marketing budgets and more precise targeting, ensuring campaigns reach the right audience with maximum impact.
Customer feedback is a goldmine for insights, and integrating it into analytics systems creates a feedback loop that fosters adaptive learning. By analyzing qualitative data from surveys and reviews and supporting interactions alongside quantitative metrics, businesses gain a holistic view of customer sentiment. Tools like natural language processing (NLP) can categorize feedback into actionable themes, such as product quality or service issues. This information informs iterative changes, such as product adjustments or improved customer support, enhancing the overall experience.
COAX stands out as a trusted partner in digital product design and development, offering expertise in creating innovative solutions that address the unique challenges of modern retail businesses. By focusing on personalized customer retention strategies, COAX ensures that retailers can stay ahead in a competitive landscape.
COAX’s retail software development services are built to meet the dynamic needs of the industry, integrating cutting-edge technology with a customer-centric approach. From designing robust eCommerce platforms to implementing advanced data analytics tools, COAX empowers retailers to create seamless and impactful customer experiences. For businesses aiming to elevate their retention strategies and maximize growth potential, COAX provides the expertise and innovation to make it happen.